Yukiyasu Kamitani
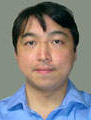
Speaker of Workshop 3
Will talk about: Decoding visual perception from human brain activity
Head of Department of Neuroinformatics at ATR Computational Neuroscience Laboratories, Kyoto, Japan, and Associate Professor at Nara Institute of Science and Technology (NAIST). He received B.A. in Cognitive Science from University of Tokyo in 1993, M.S. in Philosophy of Science from University of Tokyo in 1995, and Ph.D. in Computation and Neural Systems from California Institute of Technology in 2001. He continued his research in cognitive and computational neuroscience as a research fellow at Beth Israel Deaconess Medical Center (Harvard Medical School), and as a research stuff member at Princeton University. In 2004, he joined ATR Computational Neuroscience Laboratories, where he currently works on neural decoding of human neuroimaging signals. He was named Research Leader in Neural Imaging on the 2005 “Scientific American 50.”
Objective assessment of mental experience in terms of brain activity represents a major challenge in neuroscience. Despite its wide-spread use in human brain mapping, functional magnetic resonance imaging (fMRI) has been thought to lack the resolution to probe into putative neural representations of perceptual and behavioral features, which are often found in neural clusters smaller than the size of single fMRI voxels. As a consequence, the potential for reading out mental contents from human brain activity, or ‘neural decoding’, has not been fully explored. In this talk, I present our recent work on the decoding of fMRI signals based on machine learning-based analysis. I first show that visual features represented in ‘subvoxel’ neural structures can be decoded from ensemble fMRI responses, using a machine learning model (‘decoder’) trained on sample fMRI responses to visual features. Decoding of stimulus features is extended to the method for ‘neural mind-reading’, which predicts a person's subjective state using a decoder trained with unambiguous stimulus presentation. Various applications of this approach will be presented including fMRI-based brain-machine interface. We next discuss how a multivoxel pattern can represent more information than the sum of individual voxels, and how an effective set of voxels for decoding can be selected from all available ones. Finally, a modular decoding approach is presented in which a wide variety of contents can be predicted by combining the outputs of multiple modular decoders. I demonstrate an example of visual image reconstruction where binary 10 x 10-pixel images (2^00 possible states) can be accurately reconstructed from a singe-trial or single-volume fMRI signals, using a small number of training data. Our approach thus provides an effective means to read out complex mental states from brain activity while discovering information representation in multi-voxel patterns.