Hagai Lalazar
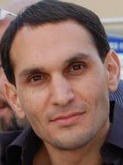
Speaker of Workshop 3
Will talk about: Sensorimotor control and learning using a Brain-Machine Interface
Hagai is currently a Ph.D. student in the lab of Prof. Eilon Vaadia, at the Hebrew University of Jerusalem. In his research they are studying the encoding properties of neurons in motor cortex and their relations to the neuronal correlations, during the planning and execution of reaching movements. To this end, they are using chronically implanted electrode arrays in behaving monkeys, trained to perform reaching tasks in a 3D virtual reality environment, as well as by using a Brain Machine Interface.
Brain Machine Interface (BMI) is being developed as a therapeutic application to restore motor control to immobilized patients. Neural signals recorded in real-time from the subject’s brain are fed into an algorithm which attempts to decipher their motor intentions and convert them into control commands that can guide various devices.
We developed a BMI environment that utilizes an adaptive version of the Kernel Auto-Regressive Moving Average (KARMA) algorithm. This method achieves high accuracy in decoding real arm movements in the offline setting, and smooth, natural-like, and successful movements in the closed-loop paradigm. Moreover, the learning algorithm allows the BMI to be used successfully practically instantly, needs no explicit training, and continually adapts in the background to any changes in neural encodings or recording quality.
In addition to its primary clinical purpose, we are using the BMI setup as a new experimental tool to study sensorimotor control and learning. The advantage of BMI is that it creates a causal and controlled direct link between a sub-population of neurons (all of which are recorded) and the behavioral output. In order to probe the encoding properties of motor cortical neurons, we compared the neural activity of the same neurons when monkeys performed the same task with either real arm movements or the closed-loop BMI. Thus the monkeys had identical motor intentions and similar cursor kinematics in both cases, yet controlled very different effectors. To investigate how such tuning properties initially develop we then let the monkeys learn to control a novel visuomotor task that they had never controlled before with their bodies, over several days. I will show how the population response slowly formed along the several days of learning.